The Unseen Dangers of AI Hallucinations: Why Tech Needs to Take It Seriously in 2025
As generative AI models evolve, so do their tendencies to hallucinate. Here's why developers, startups, and enterprises can't afford to ignore it.
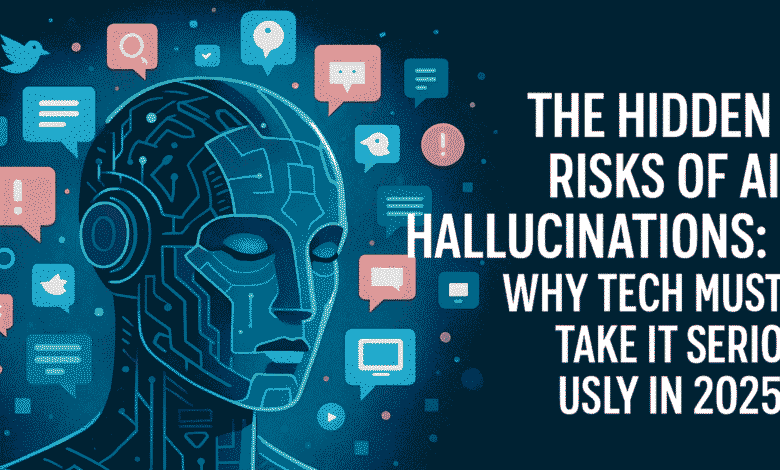
The Hidden Risks of AI Hallucinations: Why Tech Must Take It Seriously in 2025
AI is writing code, composing emails, summarizing legal documents, and creating reports in fractions of a second. But here’s the catch: sometimes it makes things up.
These misleading or untrue outputs—termed “AI hallucinations”—are more than just technical problems. In 2025, there are urgent problems that erode trust, impair compliance, and pose risks to safety.
What is An AI Hallucination?
When a generative AI model, such as GPT or Claude, confidently generates something factually wrong or completely made up, that is a hallucination. It may reference non-existent articles, manufacturing data points, or misread plain prompts in unexpected ways.
In informal use, hallucinations can be entertaining. In business or scientific contexts, however, they can lead to poor decisions, corrupted data, and reputational harm.
Why Are Hallucinations a Problem in 2025?
AI use is at a record high in finance, law, medicine, education, and government. Organizations integrate LLMs (Large Language Models) into mission-critical operations now. That is to say that a hallucinated diagnosis, legal clause, or financial projection can have tangible outcomes in the real world.
Real-world examples are piling up.
- A law firm filed a brief that included phony court cases produced by artificial intelligence, and was penalized.
- AI-generated health advice inaccurately represented published research in clinical summaries.
- AI-generated internal business reports contained fictional figures and charts.
The increasing prevalence of auto-generated material that is not manually checked is making the risk of hallucinations more common and more expensive.
Why Hallucinations Occur
Hallucinations arise from text predictions of generative models. These models aim to be plausible-sounding rather than truthful unless fact-checked with live facts in the moment. Even with reinforcement and fine-tuning, models continue to take reasoning leaps and err in response to esoteric, vague, and multi-step ideas.
What Can Be Done?
- Retrieval-Augmented Generation (RAG): Integrate AI with search in real-time to minimize fabrication
- Human-in-the-Loop Review: Always include human review of high-risk outputs
- Learning from Verified Datasets: Utilize hand-crafted, specialized datasets
- Confidence Scoring: Make users understand when an output is not reliable
- Transparent Model Design: Define limitations and boundaries of the model at the beginning
Closing Thoughts
AI hallucinations aren’t merely flaws. They’re indicators of just how much we still require human judgment, design guardrails, and accountability in machine systems.
With increasing infusion of AI in our lives and institutions, recognizing and avoiding hallucinations has to be every AI roadmap’s component.